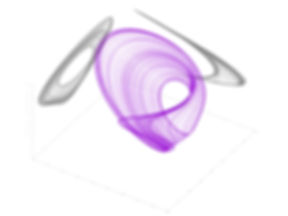
DECIPHer - Selected applications
The research carried-out in the team is by-essence multi-disciplinary and lies at the interface between computational mechanics, applied mathematics, statistics, dynamical systems, machine learning, etc. Our desire is to apply our methodologies on large-scale systems through several real applications. Recent examples of our work include:
- Uncertainty quantification and propagation, sensitivity analysis and data assimilation in compressible flows, fluid-structure interactions, turbulent environmental flows, hemodynamics, etc.
- Low-dimensional models assisting Direct Numerical Simulations (DNS). For instance, the velocity field near solid walls in a turbulent flow simulation is described by a low-dimensional model, typically a modal-based (e.g., POD) reduced-order model or a neural network, interfacing with the Navier-Stokes simulation of the bulk flow. It results in a hybrid model involving significantly fewer degrees-of-freedom than the standard DNS.
- Field reconstruction from limited sensors. An observer is taught how to link sensor measurements to the full field description. This situation typically applies when one wants to estimate the state of a system from partial observations.
- Prediction and Reinforcement Learning for the control of chaotic systems. A predictive model and a control policy are learned from data to minimize a cost function through interactions with the environment of the system. Applications to dynamical systems and experimental flows.